Software
Jump to:[χ-CNN|CNEP|CSREP|ChromGene|ChromHMM|ChromTime|ChromImpute|ConsHMM|DREM|LECIF|SEREND|SHARPR|STEM]
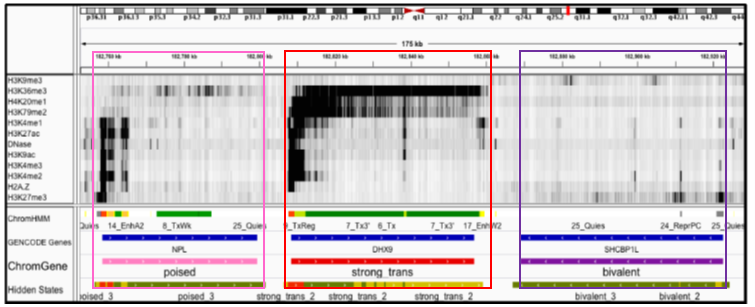
ChromGene is a method for gene-based modeling of epigenomic data.
Citation:
Jaroszewicz A, Ernst J.
ChromGene: gene-based modeling of epigenomic data.
Genome Biology, 24:203, 2023.
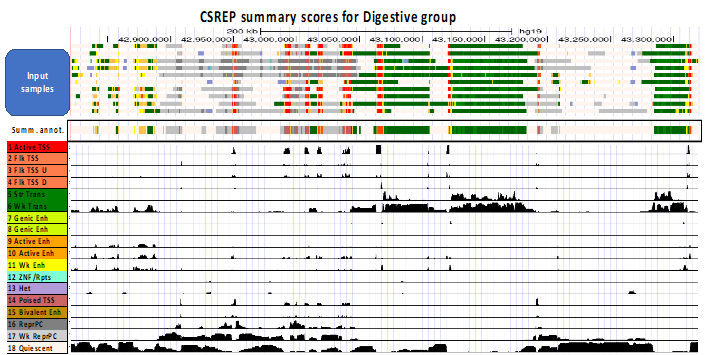
CSREP is a framework for group-wise summarization and comparison of chromatin state annotations.
Citation:
Vu H, Koch Z, Fiziev P, Ernst J.
A framework for group-wise summarization and comparison of chromatin state annotations.
Bioinformatics, 39:btac722, 2023.
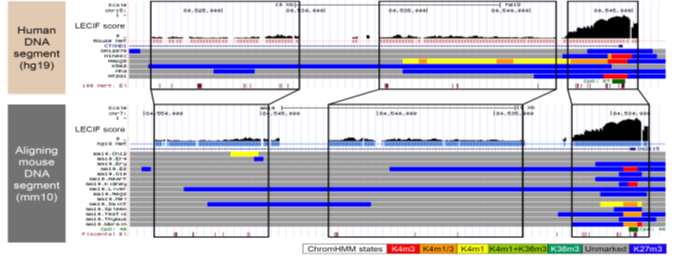
LECIF is a supervised machine learning method that learns a genome-wide score of evidence for conservation at the functional genomics level.
Citation:
Kwon SB, Ernst J.
Learning a genome-wide score of human-mouse conservation at the functional genomics level.
Nature Communications, 12:2495, 2021.

CNEP is software for predicting constrained-non exonic bases from large scale epigenomic and transcription factor binding data.
Citation:
Grujic O, Phung TN, Kwon SB, Arneson A, Lee Y, Lohmueller KE, Ernst J.
Identification and characterization of constrained non-exonic bases lacking predictive epigenomic and transcription factor binding annotations.
Nature Communications, 11:6168, 2020.
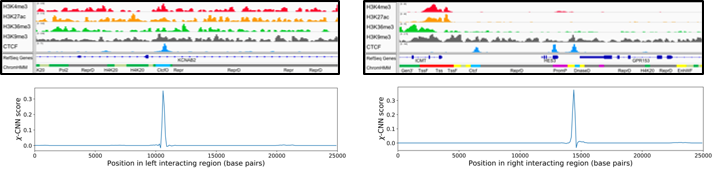
χ-CNN is software that integrates epigenomic and transcription factor binding data with more coarsely mapped chromatin interactions to fine-map the most likely sources of interactions.
Citation:
Jaroszewicz A, Ernst J.
An Integrative Approach for Fine-Mapping Chromatin Interactions.
Bioinformatics, 36:1704-1711, 2020.

ConsHMM is software for discovering conservations states and annotating genomes at single nucleotide resolution based on them.
Citation:
Arneson A, Ernst J
Systematic discovery of conservation states for single-nucleotide annotation of the human genome.
Communications Biology, 248, 2019.
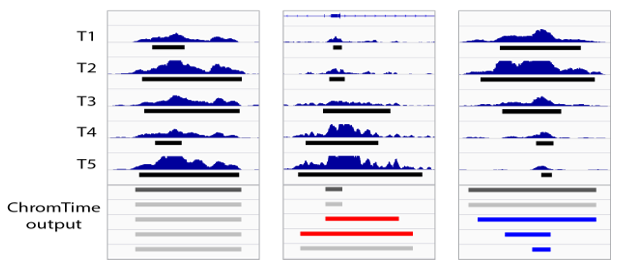
ChromTime is software for modeling the spatio-temporal dynamics of chromatin marks over time allowing systematic detection of expansions, contractions, and steady regions of chromatin marks over time.
Citation:
Fiziev P, Ernst J
ChromTime: modeling spatio-temporal dynamics of chromatin marks.
Genome Biology, 19:109, 2018.
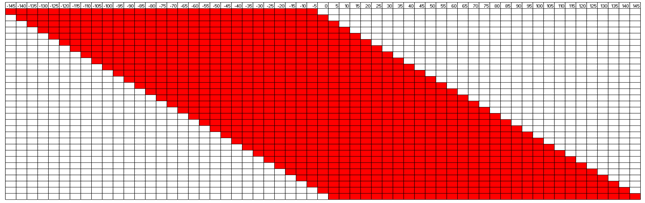
SHARPR is software for analyzing Massively Parallel Reporter Assay tiling designs allowing mapping at high resolution activating and repressive nucleotides across thousands of regulatory regions.
Citation:
Ernst J, Melnikov A, Zhang X, Wang L, Rogov P, Mikkelsen T, Kellis M.
Genome-scale high-resolution mapping of activating and repressive nucleotides in regulatory regions.
Nature Biotechnology, 34:1180-1190, 2016.

ChromImpute is software for large-scale systematic epigenome imputation. ChromImpute takes an existing compendium of epigenomic data and uses it to predict signal tracks for mark-sample combinations not experimentally mapped or to generate a potentially more robust version of data sets that have been mapped experimentally. ChromImpute bases its predictions on features from signal tracks of other marks that have been mapped in the target sample and the target mark in other samples with these features combined using an ensemble of regression trees.
Citation:
Ernst J, Kellis M.
Large-scale imputation of epigenomic datasets for systematic annotation of diverse human tissues.
Nature Biotechnology, 33:364-376, 2015.
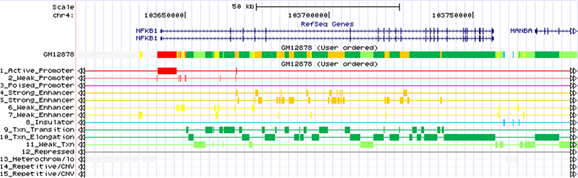
ChromHMM is software for learning and characterizing chromatin states. ChromHMM can integrate multiple chromatin datasets such as ChIP-seq data of various histone modifications to discover de novo the major re-occuring combinatorial and spatial patterns of marks. ChromHMM is based on a multivariate Hidden Markov Model that explicitly models the presence or absence of each chromatin mark. The resulting model can then be used to systematically annotate a genome in one or more cell types. By automatically computing state enrichments for large-scale functional and annotation datasets ChromHMM facilitates the biological characterization of each state. ChromHMM also produces files with genome-wide maps of chromatin state annotations that can be directly visualized in a genome browser.
Citation:
Ernst J, Kellis M.
ChromHMM: automating chromatin-state discovery and characterization.
Nature Methods, 9:215-216, 2012.
Papers using ChromHMM


The Dynamic Regulatory Events Miner (DREM) allows one to model, analyze, and visualize transcriptional gene regulation dynamics. The method of DREM takes as input time series gene expression data and static or dynamic transcription factor-gene interaction data (e.g. ChIP-seq, ChIP-chip data), and produces as output a dynamic regulatory map. The dynamic regulatory map highlights major bifurcation events in the time series expression data and transcription factors potentially responsible for them.
Citation:
Ernst J, Vainas O, Harbison CT, Simon I, and Bar-Joseph Z.
Reconstructing dynamic regulatory maps.
Nature-EMBO Molecular Systems Biology, 3:74, 2007.
Papers using DREM

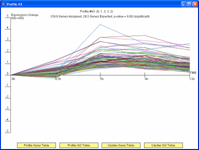

The Short Time-series Expression Miner (STEM) is a Java program for clustering, comparing, and visualizing short time series gene expression data from microarray experiments (~8 time points or fewer). STEM allows researchers to identify significant temporal expression profiles and the genes associated with these profiles and to compare the behavior of these genes across multiple conditions. STEM is fully integrated with the Gene Ontology (GO) database supporting GO category gene enrichment analyses for sets of genes having the same temporal expression pattern. STEM also supports the ability to easily determine and visualize the behavior of genes belonging to a given GO category or user defined gene set, identifying which temporal expression profiles were enriched for these genes. (Note: While STEM is designed primarily to analyze data from short time course experiments it can be used to analyze data from any small set of experiments which can naturally be ordered sequentially including dose response experiments.)
Citation:
Ernst J, Bar-Joseph Z
STEM: a tool for the analysis of short time series gene expression data.
BMC Bioinformatics, 7:191, 2006.
Papers using STEM

The SEmi-supervised REgulatory Network Discoverer (SEREND) is a semi-supervised learning method that uses a curated database of verified transcriptional factor-gene interactions, DNA sequence binding motifs, and a compendium of gene expression data in order to make thousands of new predictions about transcription factor-gene interactions, including whether the transcription factor activates or represses the gene.
Citation:
Ernst J, Beg QK, Kay KA, Balazsi G, Oltvai ZN, Bar-Joseph Z.
A Semi-Supervised Method for Predicting Transcription Factor-Gene Interactions in Escherichia coli.
PLoS Computational Biology 4: e1000044, 2008.